Introduction
The legalization of name, image, and likeness (NIL) and the "one time transfer rule" has caused major changes in collegiate athletics over the past couple of years. In large part due to these changes, the rising number of transfers has led to the transfer portal being one of the most important recruiting factors for college basketball teams to monitor. Whether a team is looking for a roster overhaul with a new coach, or looking to find the last missing piece to fill out their roster, the transfer portal provides potential solutions to traditional problems in roster construction. Unfortunately, not all transfers turn into success stories at their new schools. It takes great effort, skill, and a little bit of luck to evaluate which transfers each year will be effective players at their new schools. I built my transfer model to use as a tool in evaluating which transfers will be effective players at their new schools. I will go into a lot of detail about my model and process throughout this page, but for now I will start by describing the data I used.
To create an effective transfer model, I first needed to acquire data on which players were transferring, where they were transferring to, their stats for the year before transferring (to use as feature data), information about their season after transferring (whether they moved to a better conference, etc.), and a measurement of performance for their year after transferring (Box Plus Minus). The post transfer season BPM is the "target" data that I attempt to predict. I acquired the data about transfers and where they were transferring to from the verbal commits website, and I acquired all the player stats from basketball reference. After cleaning and merging the data, I was able to construct a dataset that contained a player's stats, transfer information, pre transfer performance, and post transfer performance. An example of what this data looks like can be seen in Figure 1. I'll go into more detail about what some of those columns mean later on if there is any confusion, but the general gist of what the data looks like can be seen in that figure. One important thing to note is that only players who played 75% of their team's games in both their pre transfer AND post transfer season are included in the data. This criteria was established so that there was a sufficient sample size for each player.
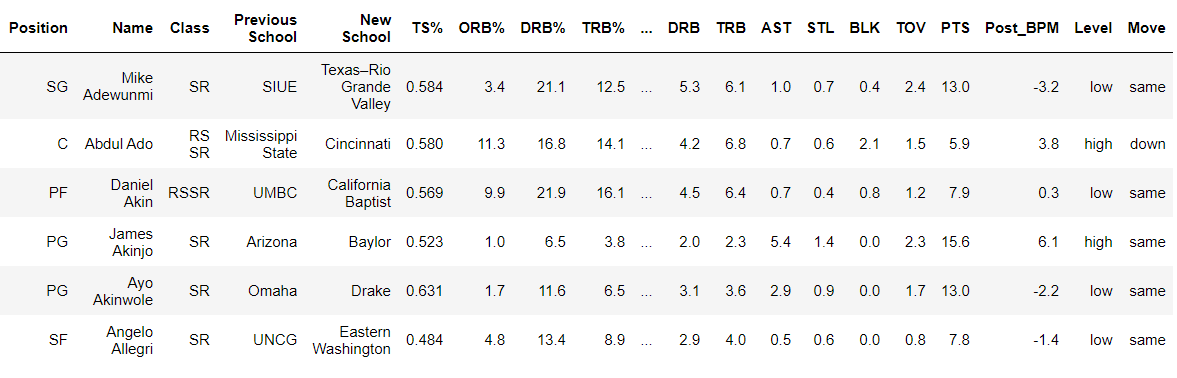
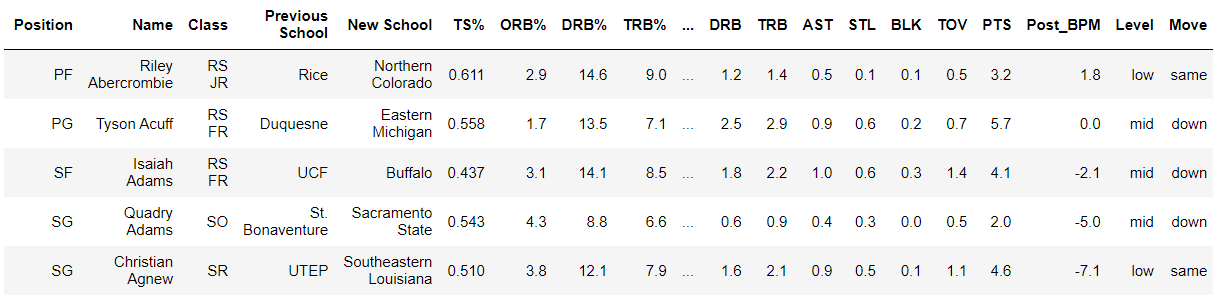
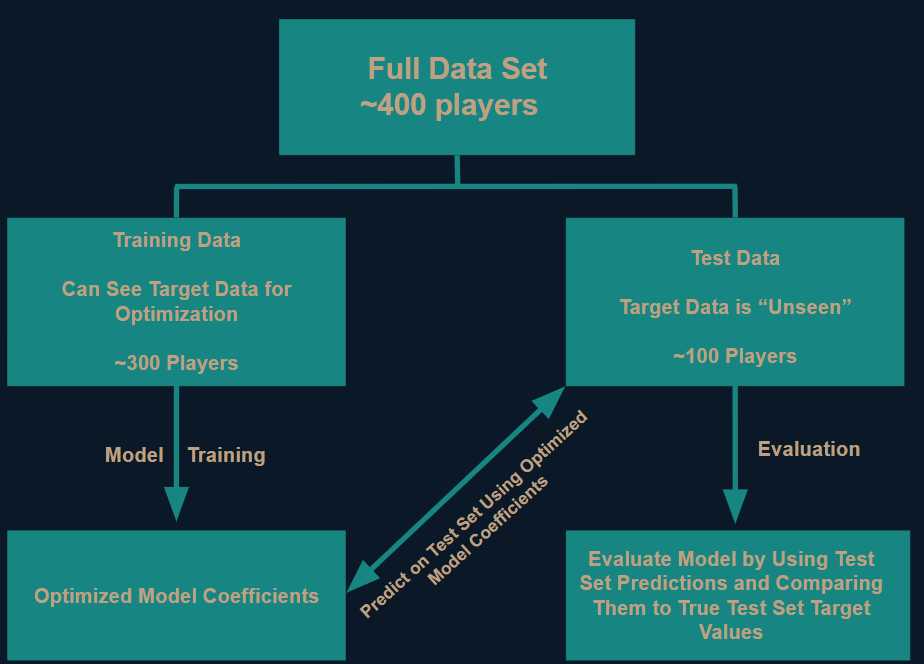
Method
Before I could get into the actual modeling, I created two categorical features called “level” and “move”. The feature “level” was created to hold information on the level of competition a player was playing at before transferring. The categories of level are “high” (Big 10, Big 12, SEC, ACC, Pac 12, Big East), “mid” (WCC, Mountain West, AAC, A10), and “low” (the rest of the conferences). I decided to categorize each conference according to how many teams the conference consistently sends to the NCAA Tournament. Low major conferences are one bid leagues, while mid major conferences typically send between two and four teams to the tournament. High major conferences consistently send four or more teams to the tournament. The feature “move” takes each player's pre transfer conference and post transfer conference into account and categorizes the change in competition as one of the following five options: “2 down”, “down”, “same”, “up”, “2 up”. To provide an example, Justin Ahrens played at Michigan State (Big 10) in the 2021-22 season and transferred to Loyola Marymount (WCC) for the 2022-23 season. For Justin's case, he would be given the “high” (Big 10) categorization for the “level” feature and a “down” (high to mid) categorization for the “move” feature.
I used the dataset with player stats from the 2020-21 season to train a model to predict each player's post transfer season BPM (2021-22 season in this case). To do this, I performed a randomized 75%/25% train/test split. In other words, I randomly took 75% of the data (about 300 players) as a training set, and I trained several different models to predict the player's post transfer BPM. These models were able to see the actual post transfer BPM in order to optimize the coefficients for each feature. Next, I used the models that I just trained to make predictions on the remaining 25% of the data. This remaining test set contained “unseen” data, as the models were not able to see the true post transfer BPM like they could with the training set. This allowed me to evaluate how good the model was by taking the predicted values and seeing how close they were to the actual post transfer BPM values. Figure 2 illustrates this process.
Model Pre-Processing
For these models to be as effective as possible, I needed to perform some pre-processing steps so that my models could understand the data. I used Scikit-Learn's “Column Transformer” to make the necessary modifications. For the “level” and “move” columns I encoded each category as a 1 if that category described the player and 0 otherwise. This led to the creation of eight new columns: one for each of the three categorizations of “level” and one for each of the five categorizations of “move”. For example, Justin Ahrens would have a value of 1 for the columns “high” and “down”, and a value of 0 for the remaining six categorization columns. This encoding was necessary because the models can only understand numerical data, and “level” and “move” are categorical columns.
The other preprocessing step I needed to do was to “standardize” the numerical value columns. What this means is that I transformed the columns to reflect how much more or less feature values were than their feature average, while taking into account the standard deviation. This is important because the scales of each column vary. For example, a player can play 40 minutes a game (MPG feature) while producing 100 points on the season (PProd feature). In a raw sense, 100 is much larger than 40; however, compared to the average, 40 minutes per game is well above average, while 100 points produced is well below average. Certain kinds of models are not able to account for different scales, so by comparing each feature value to its average value (and accounting for each feature's standard deviation), you force all the features onto the same scale. You can read here for more details on standardization.
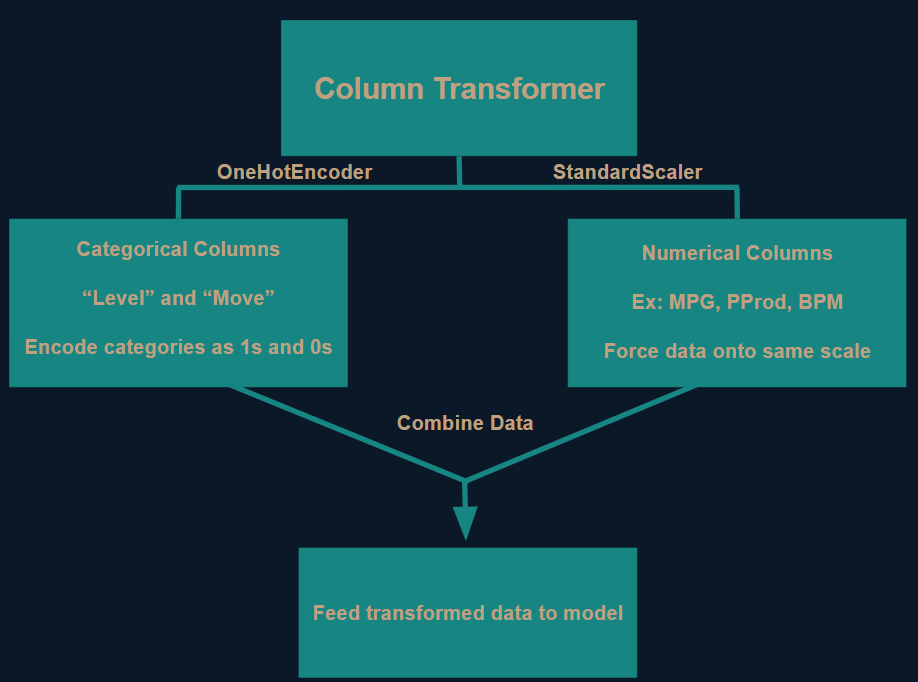
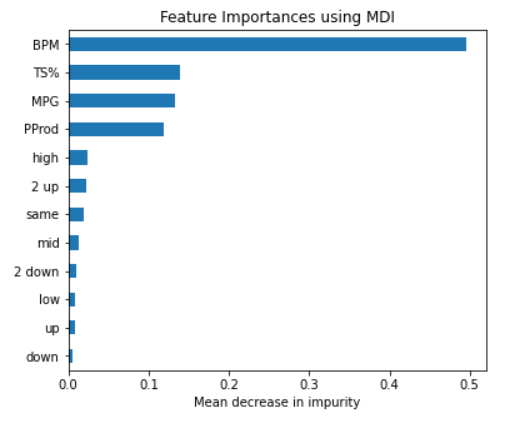
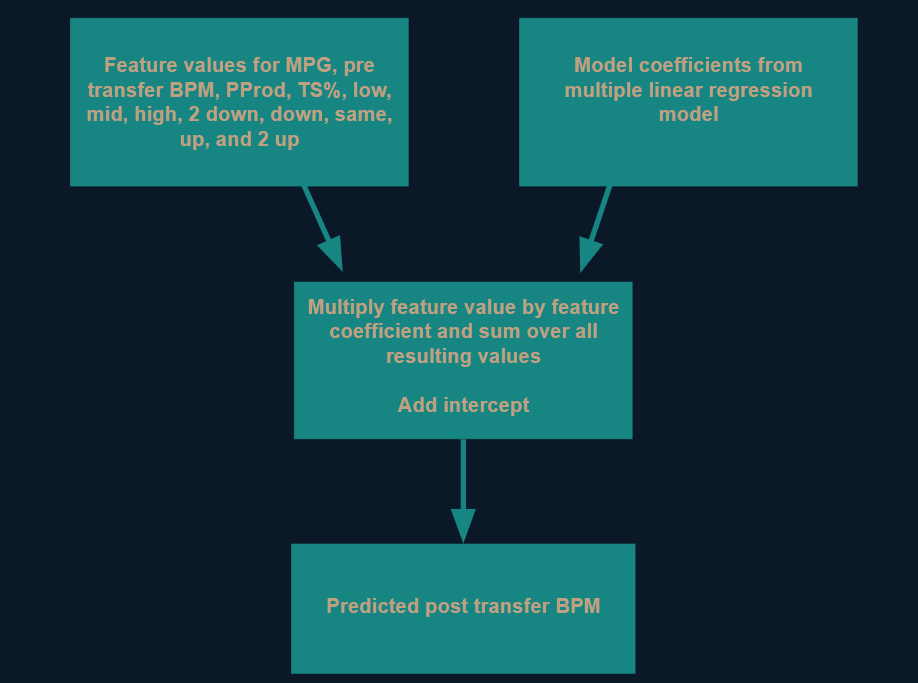
Model Results
After creating the features “level” and “move” and applying the appropriate pre-processing steps to the data, I selected which features to use in the models. I performed several rounds of model testing and feature exploration to narrow down which features appeared to be the most important. I then explored different combinations of those features and examined their impact on model score to get to the final few possibilities. In the end, I decided to use the following features for my models: minutes per game, pre transfer BPM, points produced, true shooting percentage, low, mid, high, 2 down, down, same, up, and 2 up. The last eight of those features are the encoded category values of the “level” and “move” features. There were some different feature combinations that provided marginal model score improvements that only included “down”, “2 down”, and “high” out of the eight encoded features. I decided to include them all despite the very slight decrease in score as I believed that including all of them increased the interpretability and made for a more intuitive model. Figure 4 provides a visual of the feature importance of the final combination of features that I used for my model. Pre transfer BPM was clearly the most important feature, but as I will detail in the next paragraph, adding the other features led to a substantial improvement in model score. Another thing to note is that each of the eight encoded features by themselves are not very important, but taking them all together does make an impact on the model.
As I mentioned previously, I trained multiple different kinds of models as I wanted to see which one was most effective for this data set. I tested several kinds of regression models such as multiple linear regression and ridge regression as well as a couple ensemble methods such as random forest regression and gradient boosting regression. In the end, the multiple regression model proved to be the most effective method for modeling this data regardless of what features were used. For the final multiple linear regression model I used, I was able to achieve an R-squared value of .406. This means that my model was able to explain almost 41% of the variation in post transfer BPM. While obviously I would like that percentage to be as close to 100% as possible, 41% is quite good considering all the variables at play that are not quantifiable. Factors such as moving to a new place, playing in a new system, offseason skill improvement (or decline), and numerous other things all contribute to how well a player plays after transferring. Additionally, this model served as a clear improvement over the baseline model that I created which only used pre transfer BPM to predict post transfer BPM. This baseline model had an R-squared value of .255. My model was able to explain over 15% more of the variation in post transfer BPM than the baseline model could. The random forest model also outperformed the baseline model with an R-squared score of .313, but this model was not able to produce as effective results as the multiple regression model.
Predictions for the 2022-23 Season
I applied my final model to the dataset with the 2021-22 pre transfer season data to generate the 2022-23 post transfer predictions.
*Players must have played 75% of their teams games in both the 2021-22 and 2022-23 season to be included.*
*Some players may be exluded to due to inconsistencies when merging data from different sources.*
Model Accuracy
After the completion of the 2022-23 NCAA basketball season, it is time to go back and evaluate my transfer predictions. As a reminder, the goal of this model is to identify “effective” college basketball transfers. What exactly “effective” means is subjective, but for this case I've decided to evaluate an effective transfer as one who has matched or exceeded a BPM value of two. I'm choosing this benchmark because I believe that outscoring an opponent by two more points per 100 possessions compared to an average producer qualifies you as an “effective” player. Once again, there are many valid opinions on what makes an effective player, and many of those pay no consideration to BPM. With that being said, BPM provides a quality advanced statistic that can serve as a useful benchmark for evaluation.
Now for the question of how accurate my model was in predicting effective transfers. Of the 130 transfers that my model predicted to have a BPM value greater than or equal to 2, 93 of them actually did. In other words, for every four transfers my model predicted to be “effective”, about three of them actually were (~72% accuracy).
Additionally, of those 37 errors, only 17 of them ended up being negative BPM players, meaning that the team was worse with that player on the floor compared to the average player production. This means that approximately 87% of the time my model predicted a player to be an “effective” transfer, they were at least not a negative contributor. With how many variables go into a player's impact on their new team after transferring, I would say that the model did a strong job of accurately predicting effective transfers this year. In terms of errors going the other way around (predicted to NOT be effective but actually were) my model was also very accurate. Approximately 81% of the time a player was predicted to have a BPM less than 2 by my model, they actually did. With that being said, this kind of error is not as impactful as the first case. If you are expecting someone to be an effective player and they aren't, that is much more damaging than expecting someone to not be an effective player and being pleasantly surprised when they are.